Finding a Faster, More Accurate Way to Identify Molecular Structures of Natural Products
Roughly 70 percent of drugs approved by the U.S. Food and Drug Administration are based on natural products such as plants and microorganisms in the soil or in the ocean. Now, four researchers from the Computer Science and Engineering department are part of an interdisciplinary team from UC San Diego that led development of a new method that is significantly faster and more accurate than existing methods for identifying the molecular structures of natural products.
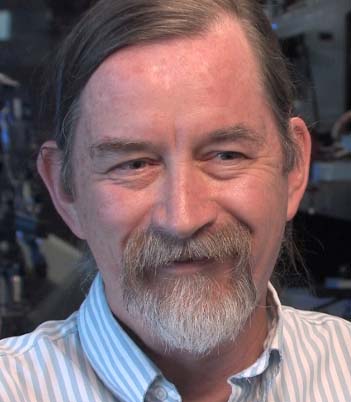
CSE professor Gary Cottrell is co-senior author on a paper* published online in the journal Nature Scientific Reports that spells out what the team calls Small Molecular Accurate Recognition Technology (SMART) and its benefits.
According to the paper’s authors, the new technique has the potential to achieve a ten-fold speed-up in the time it takes to identify a molecular structure from a natural product. As such, the SMART system could represent a new paradigm in chemical analysis and pharmaceutical drug discovery.
UC San Diego has a patent pending on the SMART technique. Named inventors on the patent application include both senior authors – CSE’s Cottrell and Scripps Institution of Oceanography professor William Gerwick – and both first authors: NanoEngineering Ph.D. student Chen Zhang (who works in Gerwick’s lab at Scripps), and CSE alumnus Yerlan Idelbayev (M.S. ’16), who worked on the project while still in CSE, but is now a Ph.D. student at UC Merced.
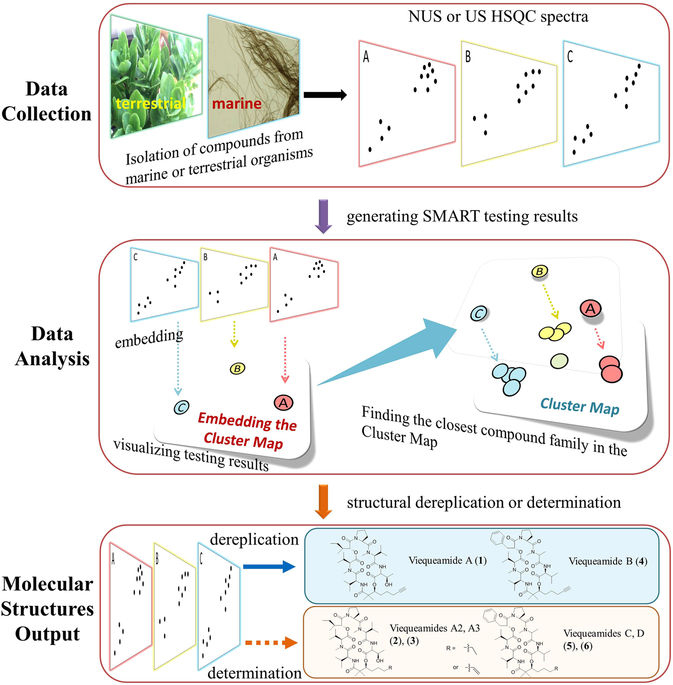
Technology (SMART).
Other CSE-affiliated co-authors of the paper include two undergraduate researchers from Prof. Cottrell’s lab who are majoring in Computer Science: junior Nicholas Roberts, who explored the effects of artificial experimental noise added to the SMART deep learning dataset; and senior Yashwanth Nannapaneni, who was a software engineering intern at Amazon over the summer. He expects to graduate next June.
In addition to the research-enabling information," said UC San Diego oceanography and pharmaceutical sciences professor Bill Gerwick, Cottrell’s co-senior author on the new study. "You have to have the structure for any FDA approval. If you want to have intellectual property, you have to patent that structure. If you want to make analogs of that molecule, you need to know what the starting molecule is. It's a critical piece of information."
The SMART method uses a piece of spectral data unique to each molecule and then runs it through a deep learning neural network to place the unknown molecule in a cluster of molecules with similar structures. "The way we were able to accelerate the process is by essentially using facial recognition software to look at the key piece of information we obtain on the molecules," said Gerwick.
The key piece of information the team uses is called a heteronuclear singular quantum coherence (HSQC) nuclear magnetic resonance (NMR). Each HSQC NMR spectrum produces a topological map of spots that reveal which protons in the molecule are attached directly to which carbon atoms, an arrangement unique to each molecule.
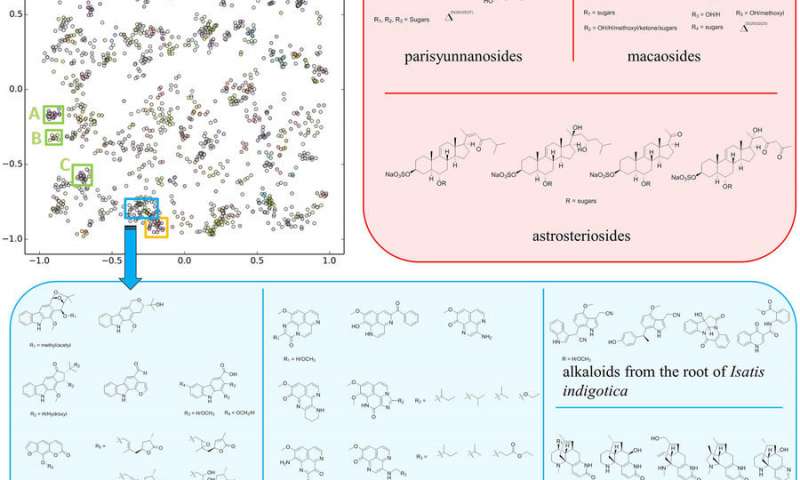
Read more here .
CSE’s Cottrell and his team then developed a deep learning system that was trained with only around 2,000 2D images of HSQC spectra compiled from prior research. The convolutional neural network (CNN) took the images of spectra of unknown molecules and mapped them into a ten-dimensional space near molecules with similar traits.
“This is normally not enough data to train a deep network, but we used a technology called a Siamese network, in which you train on pairs of images,” said Cottrell. “This amplifies your training set by roughly the square of the number of compounds in a family, and is what made this project feasible.”
According to the article, as more compounds are added to the training set, “the SMART system will naturally improve in accuracy and robustness, thereby accelerating natural product structural elucidation and thus drug discovery.”
In their Nature Scientific Reports paper, the co-authors concluded that while they looked only at certain metadata associated with the spectra used in the study, “it is very possible to associate and integrate biological, pharmacological and ecological data with SMART, and thereby create new tools for enhanced discovery and development of biologically active natural products as well as other small molecules.”
____________________________________
*Chen Zhang, Yerlan Idelbayev, Nicholas Roberts, Yiwen Tao, Yashwanth Nannapaneni, Brendan M. Duggan, Jie Min, Eugene C. Lin, Erik C. Gerwick, Garrison W. Cottrell and William H. Gerwick, Small Molecule Accurate Recognition Technology (SMART) to Enhance Natural Products Research, Nature Scientific Reports, Issue 7, Article 14243, published online 27 October 2017. (doi: 10.1038/s41598-017-13923-x)
Related Links
SMART Article in Nature Scientific Reports
PhysOrg News Article
Cottrell Lab
Media Contacts
Doug Ramsey, (858) 822-5825, dramsey@ucsd.edu